Most collections departments use A/B testing to test and learn, refining their approach based on the results. While A/B tests are great tools, they do have some downsides:
- You should generally run an A/B test for one to two weeks in order to gain a statistically representative sample;
- You then have to dedicate a significant portion of time and energy analysing the data;
- You might fall into the trap of thinking that the winner from your A/B test is the best and only option. Unfortunately, this is not always the case—different methods work best for different scenarios.
The solution is not to throw A/B tests out of your strategy entirely. Instead, you must understand their limitations and implement alternative approaches that make up for these shortcomings.
In other words, you should adopt a multi-armed bandit (MAB) approach. By doing so, you will spend less time interpreting results and putting them into action, and more time serving consumers with your most effective dunning approaches.
This article will dive into the shortcomings of A/B testing, what MAB is, and how it powers collections success.
Current hurdles that collections operational teams face
The best collections teams are continually updating and refining their strategy. However, they can run into a range of hurdles.
1. Not knowing which email templates work the best due to lack of automation
A/B testing is a statistical experiment generally conducted manually. Simply put, you challenge option A against option B, run the tests and then dig into the data once it is completed. After identifying the results you can then finally use these insights to improve your strategy.
So while the concept is fairly simple, putting the results into action is anything but effortless.
Collections teams generally lack a way to test different templates at scale, to receive the results in an instant, and to immediately leverage crucial data-driven learnings. They therefore spend more time and effort discerning which email templates work best, while also spending more time using ineffective strategies.
2. Not knowing which messaging delivery time works best for which segment
Optimising your outreach strategy requires fine-tuning a myriad of factors: the type of message, the channel, and the time you send your messages out.
But working out the precise best time to send out a dunning message is no mean feat, especially if you rely upon physical methods (such as direct mail) where you have no visibility into when your letter is actually opened.
Even if you are using digital methods, however, you still have to adopt a manual approach. This involves waiting for the results, discerning what the data is telling you, and finally, making the appropriate adjustments. Doing this at scale is difficult, time-consuming and inefficient.
3. Processing time taking too long—and not always providing a clear path
A/B tests do give you effective results, though it does require a lot of patience. In many cases, you have to work with one hand tied behind your back—you do not yet know how to segment your customers, so you might have to arbitrarily segment them according to factors that might not be all that important.
But once you start an A/B test, there is often a long processing time (though this largely depends on how much data you have and how many variants you examine). You cannot dive in midway through and change it according to the results. Testing is not so much an iterative, ongoing process, with agents refining their approach as soon as new information comes in. Instead, it is more stop-start, with there being a delay between receiving results and putting these insights into action.
A/B tests can also be further hampered by the data. Too much data renders you unsure of where to begin, leaving strategists and agents suffering from analysis paralysis. It becomes virtually impossible to identify which specific variants are actually making a difference, and which can be discarded.
Too little data, on the other hand, means that you’re left with few historical traces that can be analysed to make decisive insights and to tangibly improve your performance going forward.
How can MAB help?
Multi-armed bandit (MAB) refers to the concept of a gambler trying to work out which slot machine will produce the best results. Picture multiple arms attached to one slot machine. In the tech world, it is a machine learning algorithm, which explores the result first and applies machine learning afterwards to keep further exploiting and discovering the most optimal strategy during the operational processes.
In collections, you need to adopt a similar approach: leveraging AI and machine learning to test multiple strategies, identify which strategies or dunning templates perform the best, and invest more time/energy/resources into these strategies as opposed to their lower-performing counterparts.
If you can automatically test, refine, and prioritise multiple strategies at once, you broaden and boost your collections success. This is where the MAB approach, adopting machine learning, comes in.
How does MAB differ from A/B testing?
Unlike A/B testing, MAB is an iterative process. MAB gathers key data on an ongoing basis before automatically putting these insights into action, meaning agents can simply sit back and let the algorithm do its thing.
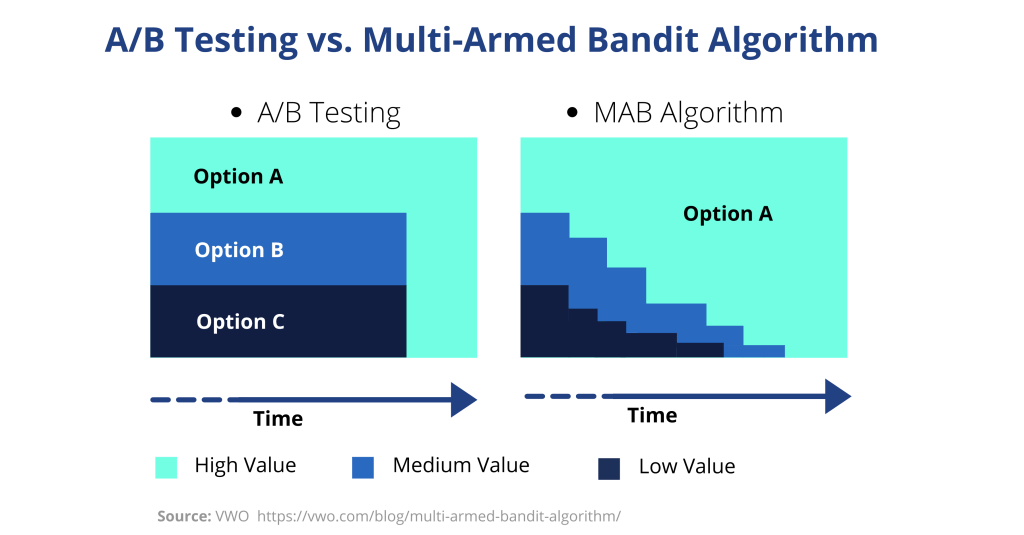
A/B tests are great for exploration (i.e testing an assumption and receiving data to help identify if this is true). MAB, however, goes one step further: prioritising both exploration and exploitation. In the beginning, it is in exploration mode. Once it begins to receive the results, however, it can then go into exploitation mode—leveraging machine learning capability to automatically apply these insights to refine your dunning approach.
This does not mean that A/B tests are redundant—far from it. They are still fantastic at helping you gather critical data-driven insights, shedding light on your customers and their behaviour. But if you want to avoid the manual process of digging into the numbers, understanding the insights they reveal, and leveraging these learning effectively, then you should consider also utilising an MAB approach.
Embrace machine learning
Machines are far better (and more efficient) than humans at crunching large datasets. They can collect, analyse, and understand thousands of data points in a matter of milliseconds. Moreover, MAB means that you can act on data-driven findings immediately—continually allocating more resources into strategies that are most effective while avoiding strategies that are not performing well.
Imagine you tried to do this manually for all your customers. Not only would it take an incredibly long time, but your agents might even be less reliable than machines. To err is human—not machine. According to Deloitte: “Using machines to analyze qualitative data could yield time and cost efficiencies as well as enhance the value of the insights derived from the data.”
Is that not the ideal scenario?
The benefits of MAB in collections
There are three primary ways in which MAB has the potential to reinvent your collections approach.
- Optimise effective messaging
MAB automatically tests different templates at once, understands which work best, and ensures these templates are prioritised going forward.
Keep manual work to a minimum. Let agents and strategists focus on simply devising a range of potential messaging templates before handing the reins over to MAB. The algorithm will explore which performs best before exploiting (i.e. favouring) those which seem to be working the best at that moment in time. If the results ever seem to change, it will then automatically allocate more resources towards those other messaging templates.
- Understand the best time to send out messages
Likewise, MAB adopts this same exploration/exploitation model to discern the best time to send out a message. It starts off with a broad array of times, sending out an equal portion of each before receiving data that highlights which times might be best. Upon receiving this data, it then prioritises successful times going forward.
- Spend more time utilising the best approaches
With MAB, you only need to focus on devising a range of approaches. Machine learning will then iteratively gather, understand, and implement data-driven learnings—allowing agents to instead spend more time with high-risk customers who need human-to-human interactions.
Increased collections success with decreased effort. The perfect blend.
MAB: your secret weapon to continued collections success
A/B tests are a mainstay for data-driven collections teams, but they have certain limitations. They require investing plenty of time and manual effort, meaning you spend too long waiting for an optimal result to boost your collections performance.
By leveraging an MAB algorithm alongside your A/B tests, however, you can automatically iterate and fine-tune your collections approaches on an ongoing basis. Sit back and relax, safe in the knowledge that your most effective strategies are automatically being prioritised.
To learn more about how receeve uses an MAB algorithm to power collections success, have a chat with one of our team.